论文标题:Identifying viruses from metagenomic data using deep learning (基于深度学习的病毒序列识别)
期刊:Quantitative Biology
作者:Jie Ren, Kai Song, Chao Deng, Nathan A. Ahlgren, Jed A. Fuhrman, Yi Li, Xiaohui Xie, Ryan Poplin, Fengzhu Sun(孙丰珠)
发表时间:11 February 2020
DOI:10.1007/s40484-019-0187-4
微信链接:点击此处阅读微信文章
全球COVID-19新型冠状病毒肺炎疫情使人们对病毒有了前所未有的关注。病毒不仅可以侵入人体等真核生物,还可以侵入细菌等原核生物。侵入细菌的病毒数量大约有1031,是地球上最丰富的生物体 [1]。病毒入侵细菌具有很强的特异性。通过与细菌的相互作用,病毒可以控制细菌的种类和数量,从而影响微生物群落的功能。例如,人类肠道微生物中的病毒的失调会导致肠炎[2];海洋和土壤中的病毒调控着全球生物化学循环 [3]。
过去由于实验技术的限制,人类对病毒的认识只有冰山一角[4]。最新的宏基因组测序技术可以对包括病毒基因组在内的微生物基因组进行大规模测序,大大加快了病毒的研究。为了快速识别宏基因组数据中的病毒序列,美国南加州大学定量计算生物学中心孙丰珠教授课题组在Quantitative Biology期刊上发表了题为“Identifying viruses from metagenomic data using deep learning”的文章(点击文末“阅读原文”下载PDF全文),文章在该课题组2017年开发并广泛应用的VirFinder [5]基础上进一步发展了一个基于深度学习识别病毒序列的方法-DeepVirFinder。 此方法利用了深度学习和大数据的优势,无需与参考序列比对,显著提高了病毒识别的速度和准确性,将有助于在宏基因组学时代下对病毒的研究。

文章概要
作者开发了一个基于深度学习识别病毒序列的方法-DeepVirFinder。对基因序列搭建了基于卷积神经网络(convolutional neural networks)的模型,利用大量已知的病毒序列和细菌序列进行训练,得到了最优的二元分类器(图1)。卷积神经网络的优势在于它可以自主学习得到病毒的特征(motifs),无需事先定义,因此比传统的机器学习方法更加准确。另外,此模型利用已知序列学到了病毒的一般性特征,因此比基于序列比对的传统方法在识别未知病毒上更加灵活有效。
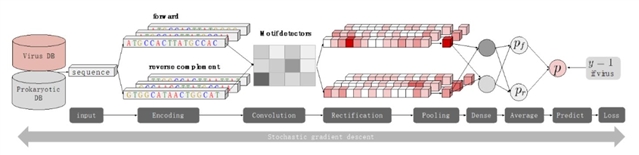
图1. DeepVirFinder的深度学习框架
DeepVirFinder模型用来自2015年5月之前发现的病毒序列进行训练,并对该日期之后发现的序列进行评估。结果显示DeepVirFinder在不同序列长度上均优于VirFinder [5],对于300、500、1000和3000 bp序列AUROC达到 0.93、0.95、0.97和0.98 (图2A)。为了进一步提高对罕见病毒识别的准确性,作者从宏基因组样本中收集了数百万条病毒序列,扩大了训练数据集(图2B)。并将DeepVirFinder应用到肠癌患者的肠道宏基因组样本中,发现了属于175个组的51138条病毒序列,其中10个组与癌症相关,表明病毒可能在肠癌中起重要作用。

图2.(A)DeepVirFinder在不同序列长度上均优于VirFinder。(B)利用从宏基因组中收集到的数百万条病毒序列扩大数据集后,罕见病毒的准确性显著提高。
摘要
Background: The recent development of metagenomic sequencing makes it possible to massively sequence microbial genomes including viral genomes without the need for laboratory culture. Existing reference-based and gene homology-based methods are not efficient in identifying unknown viruses or short viral sequences from metagenomic data.
Methods: Here we developed a reference-free and alignment-free machine learning method, DeepVirFinder, for identifying viral sequences in metagenomic data using deep learning.
Results: Trained based on sequences from viral RefSeq discovered before May 2015, and evaluated on those discovered after that date, DeepVirFinder outperformed the state-of-the-art method VirFinder at all contig lengths, achieving AUROC 0.93, 0.95, 0.97, and 0.98 for 300, 500, 1000, and 3000 bp sequences respectively. Enlarging the training data with additional millions of purified viral sequences from metavirome samples further improved the accuracy for identifying virus groups that are under-represented. Applying DeepVirFinder to real human gut metagenomic samples, we identified 51,138 viral sequences belonging to 175 bins in patients with colorectal carcinoma (CRC). Ten bins were found associated with the cancer status, suggesting viruses may play important roles in CRC.
Conclusions: Powered by deep learning and high throughput sequencing metagenomic data, DeepVirFinder significantly improved the accuracy of viral identification and will assist the study of viruses in the era of metagenomics.
Reference
[1] Breitbart M, Rohwer F. Here a virus,there a virus, everywhere the same virus? Trends Microbiol. 2005;13:278–84.
[2] Norman JM, Handley SA, Baldridge MT,Droit L, Liu CY, Keller BC, Kambal A, Monaco CL, Zhao G, Fleshner P, et al.Disease-specific alterations in the enteric virome in inflammatory boweldisease. Cell. 2015;160:447–60.
[3] Kimura M, Jia Z-J, Nakayama N, AsakawaS. Ecology of viruses in soils: past, present and future perspectives. Soil SciPlant Nutr. 2008;54:1–32.
[4] Roux S, Hallam SJ, Woyke T, SullivanMB. Viral dark matter and virus—host interactions resolved from publiclyavailable microbial genomes. Elife. 2015;4:e08490.
[5] Ren J, Ahlgren NA, Lu YY, Fuhrman JA,Sun F. VirFinder: a novel k-mer based tool for identifying viral sequences fromassembled metagenomic data. Microbiome. 2017 Dec 1;5(1):69.
Quantitative Biology期刊介绍
Quantitative Biology (QB)期刊是由高等教育出版社和清华大学共同主办的全英文学术期刊,由清华大学北京信息科学与技术国家研究中心和北京大学定量生物学中心支持。QB主要刊登生物信息学、计算生物学、系统生物学、理论生物学和合成生物学的最新研究成果和前沿进展,并为生命科学与计算机、数学、物理等交叉研究领域打造一个学术水平高、可读性强、具有全球影响力的交叉学科期刊品牌。
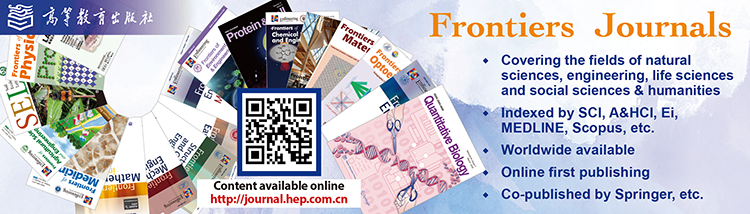
(来源:科学网)
特别声明:本文转载仅仅是出于传播信息的需要,并不意味着代表本网站观点或证实其内容的真实性;如其他媒体、网站或个人从本网站转载使用,须保留本网站注明的“来源”,并自负版权等法律责任;作者如果不希望被转载或者联系转载稿费等事宜,请与我们接洽。